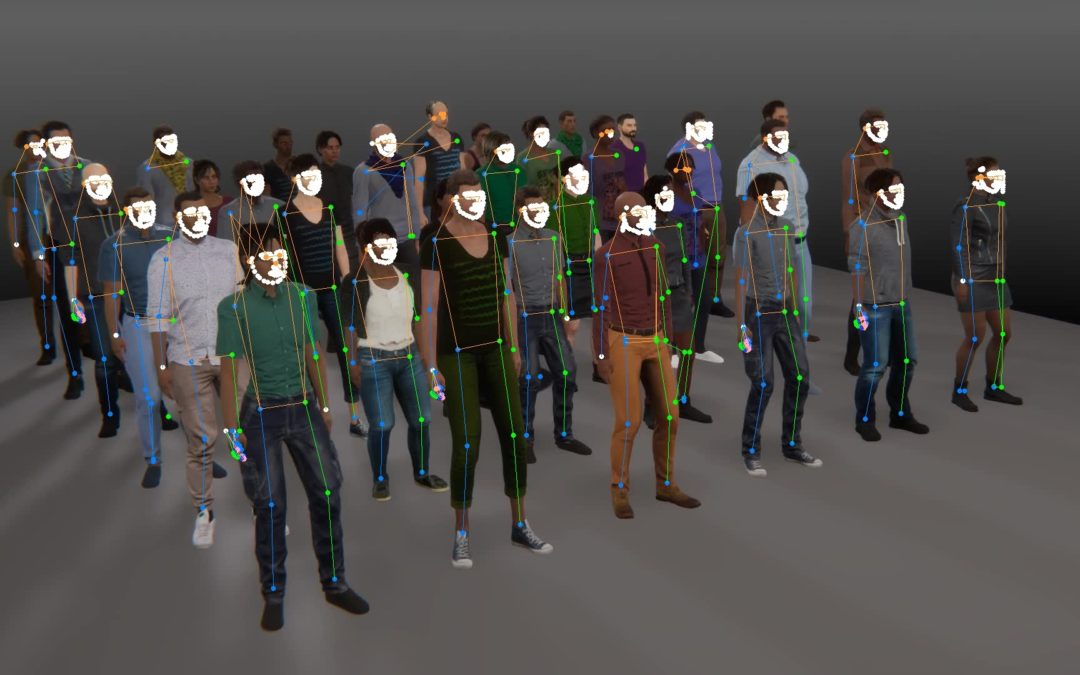
Markerless Mocap
Markerless Mocap
Killing the markersProject Info
Start date:
September 2020
End date:
September 2023
Funding:
–
Coordinator:
Artanim
Summary
Current motion capture (Mocap) solutions used in Location-based Virtual Reality (LBVR) experiences rely on a set of either active or passive infrared markers to track the user’s movements within the experience space. While this offers a stable and low-latency tracking result ideally suited for a VR scenario, equipping users with such markers is cumbersome, and the maintenance and cleaning of such devices takes time.
The Markerless Mocap project aims to leverage modern Machine Learning (ML) approaches to create a markerless solution for the capture of users in LBVR scenarios. The low-latency requirements imposed by this scenario are the primary challenge for the project, with an ideal photon-to-skeleton latency being in the order of 50ms or less. To achieve this goal the project focuses on approaches to pose-estimation which strike a good balance between accuracy and speed. The markerless pipeline consists of 3 stages: the processing of raw camera input at 60 frames per second, the 2D pose estimates of subjects in each view, and the final assembly into a full 3D skeleton. To manage this computationally heavy task at the desired latency, the overall markerless pipeline leverages the massively parallel computation abilities of modern CPUs and GPUs, allowing us to optimize every stage of the computations involved.
Another critical aspect is the training stage of any ML approach which generally requires a lot of annotated input data. And while in the field of pose estimation a variety of public datasets is available, they are often limited to a single view (where we require multi-view data for our multi-camera setups), and their annotations are not necessarily precise. Rather than record our own real-life dataset, the project opts to leverage our expertise in the creation of avatars in what we call our Synthetic Factory. Based on a single avatar model equipped with a variety of blend shapes, we can create a large variety of distinct avatars by providing broad characteristics such as age, gender, ethnicity, etc. Add in a variety of body proportions, skin tones, outfits, footwear, hairstyles, and animations, and you get a virtually unlimited set of subjects you can record from any angle, with their underlying skeleton forming the ground-truth annotation. This dataset then forms the basis of any of our training efforts.
This project was performed in collaboration with Vicon, the world leader mocap provider. First results of this joint collaboration were presented at SIGGRAPH 2023 on their exhibition booth, showcasing a six person, markerless and multi-modal real-time solve, set against Dreamscape’s LBVR adventure called The Clockwork Forest. With this showcase, Vicon earned a CGW Silver Edge Award for technological innovation.
Partners
Artanim
VR requirements definition, ML-based tracking algorithms evaluation, synthetic factory development, multi-modal tracking solution implementation and fine-tuning
Vicon
Hardware development, ML-based tracking algorithms implementation, real-time solve